DeepSeek, China, OpenAI, NVIDIA, xAI, TSMC, Stargate, and AI Megaclusters | Lex Fridman Podcast #459
The document examines how transparency in AI through open-source licensing and technical openness balances ethical, performance, economic, and geopolitical challenges while paving the way for AGI advancements.
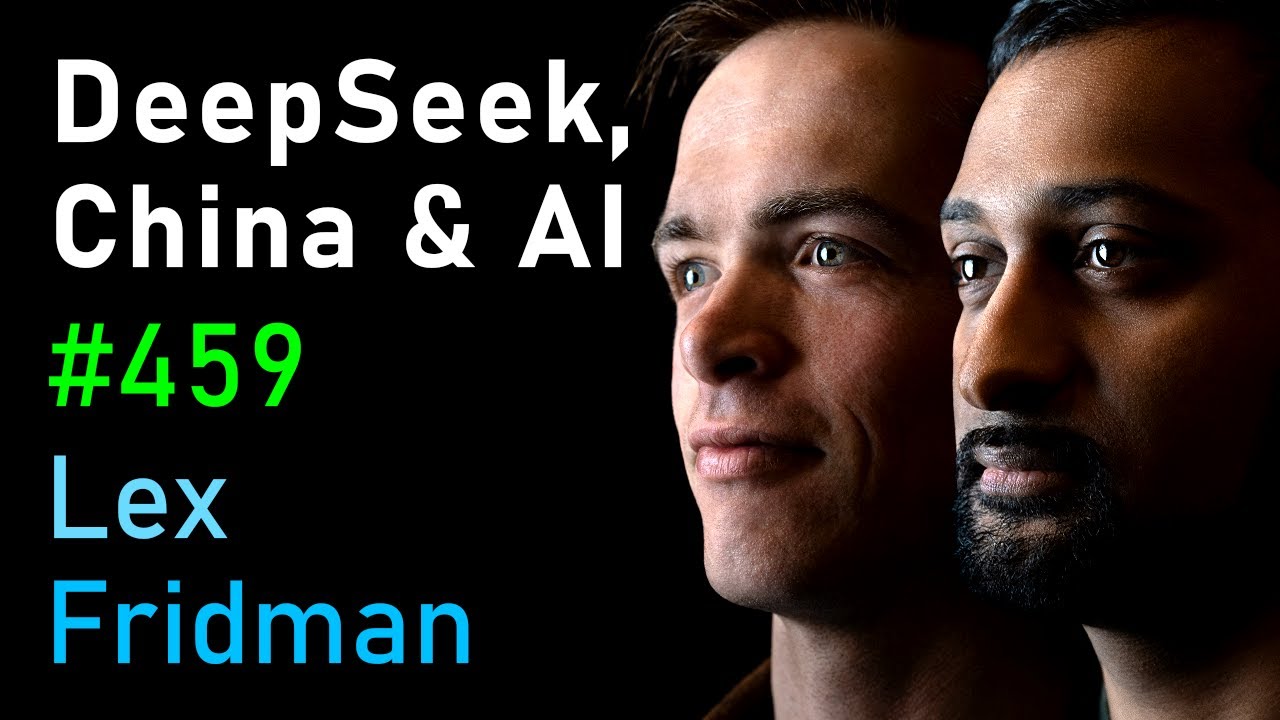
lexfridman
19 min read
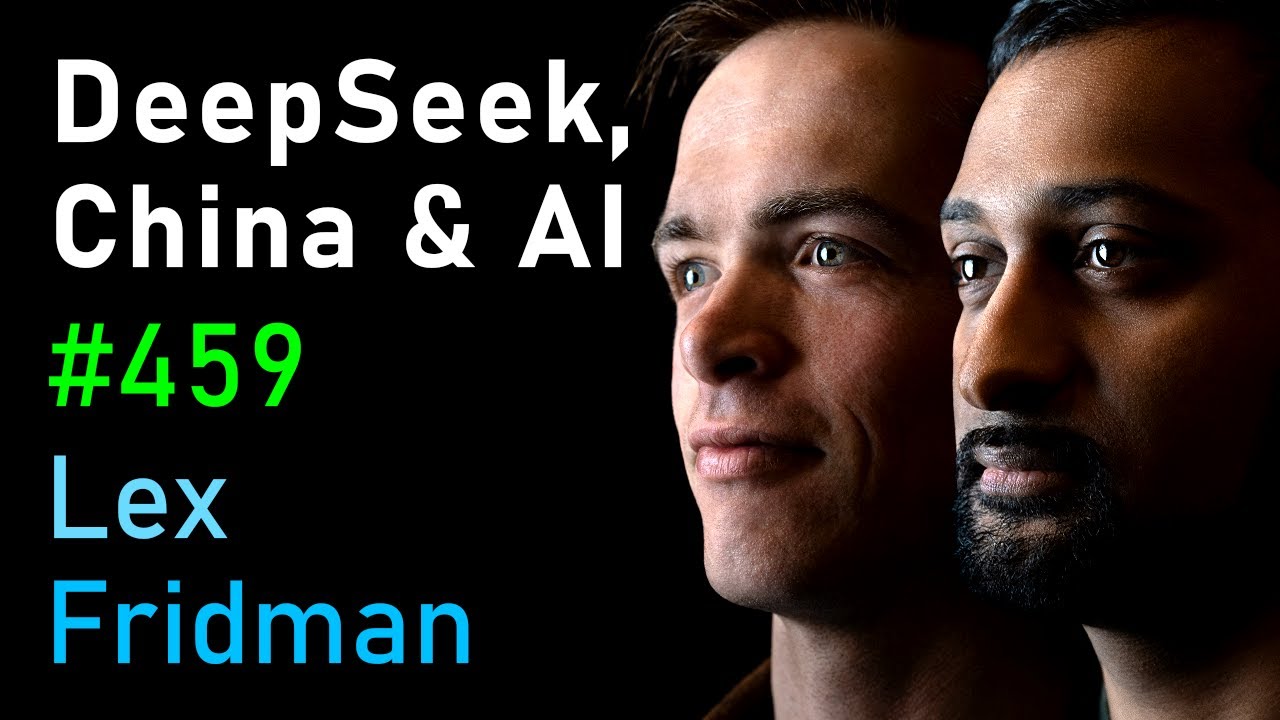
DeepSeek, China, OpenAI, NVIDIA, xAI, TSMC, Stargate, and AI Megaclusters | Lex Fridman Podcast #459
Open-Source Licensing and Open Weights: Trust and Data Control in AI Models
Open-source licensing has become a cornerstone for building trust in AI models, and the discussion highlights how open weights can empower users by placing data control firmly in their hands. For example, while some developers may worry about data theft, the conversation makes an important distinction: it isnât the model itself that presents a risk, but rather the platform on which it is hosted. DeepSeekâs approachâwith custom licenses contrasting with options like the Llama licenseâillustrates this point by offering users the ability to download and run models locally. This means that instead of entrusting sensitive, personal data to an external API, users can maintain greater control over how and where their data is processed.
The transparency inherent in open-source licensing is also emphasized by the detailed technical documentation provided by DeepSeek. By laying out specifics such as modifications at the CUDA level for Nvidia chips and interventions across different layers of the training stack, DeepSeek demonstrates a commitment not only to robust performance but also to openness about the learning process. This kind of transparency assures users that improvements are made carefully and methodically, and that data handling protocols can be independently verifiedâa significant reassurance compared to opaque, API-only models.
In an era when both American and Chinese companies are vying for dominance in AI, the ability to run models locally through open weights offers a clear strategic advantage. It helps avoid the pitfalls of having to trust a third party with one's data, underscoring the broader philosophy behind open-source practices: ensuring that users are not only participants but also guardians of their own information. In this way, open-source licensing and open weights become powerful tools in building a more secure, understandable, and user-centered AI ecosystem.
Deep Technical Transparency: Custom CUDA, Layer Interventions, and Training Process Insights
DeepSeek has distinguished itself by embracing a level of technical transparency that goes beyond the norm in AI research. The companyâs detailed documentation offers a rare glimpse into the intricate processes underlying its training regimen. For instance, DeepSeekâs reports explain how they have implemented custom modifications at the CUDA level on Nvidia chips. These tweaks arenât mere cosmetic adjustments; they are designed to optimize the training environment, ensuring that the model leverages hardware with maximum efficiency during complex computations.
In addition to these hardware-level optimizations, DeepSeek goes further by detailing specific interventions throughout the training stack. This includes well-thought-out alterations at various layers of the model, which help in fine-tuning performance and stability. By openly discussing these interventions, DeepSeek not only substantiates its claims of competing with heavyweight models like GPT-4 and Llama 405b, but also invites the broader AI community to better understandâand potentially replicateâtheir approach. The insights shared serve as a valuable resource, outlining how raw compute power is harnessed while carefully managing memory and data flow in a way that is both efficient and innovative.
Overall, this transparent approach to sharing deep technical details reinforces the importance of open scientific discourse. By demystifying aspects from custom CUDA adjustments to specialized layer interventions, DeepSeek is shedding light on the intricacies of model trainingâa move that could foster more collaborative progress across the field of AI.
Risky Breakthroughs in AI: YOLO Runs, GPU Clusters, and High-Stakes Research Gambles
Risky breakthroughs in AI often come from those high-stakes, âYOLO runâ experiments where bold bets replace incremental improvements. In these cases, researchers throw caution to the wind and unleash massive GPU clusters, betting that a single, decisive training run can lead to unexpected performance jumps. One example discussed in the text involves OpenAIâs audacious commitment in 2022 to pushing forward GPTâ4, a move seen by many as a high-risk gamble leveraging all available compute. This âYOLOâ approach contrasts with the more cautious, step-by-step optimizations pursued by other labsâwhere each small improvement builds toward a stable, predictable outcome.
This approach isnât limited to OpenAI. DeepSeek, for instance, has made headlines with its use of enormous GPU clusters to power its research and even fuel quantitative trading strategies through its affiliation with the hedge fund High-Flyer. In 2021, DeepSeek reportedly set up a 10,000 A100 GPU cluster in China, underscoring the scale and ambition of such risky investments. Rather than relying solely on gradual enhancements, these teams are willing to invest in immense computational power and cutting-edge techniques like reinforcement learning and instruction tuning during post-training phases, aiming to bridge the gap between raw performance and human-like responsiveness.
At the heart of these breakthroughs lies a dual-edged strategy: while occasional âYOLOâ experiments can spark transformative developments, they also come with significant financial and technical risks. Larger clusters, such as those envisioned by tech giants and even by innovative setups like Elonâs GPU clusters, push the limits of power consumption, cooling requirements, and interconnect speeds. This delicate balance of risk and reward has become a central theme in modern AI research, where bold gambles using high-speed, high-density hardware are often the difference between leading the field and falling behind.
In summary, the landscape of AI research is now defined by a willingness to take high-stakes research gambles. Whether itâs through risky, singular âYOLO runsâ or the deployment of mega GPU clusters that power breakthroughs in next-generation reasoning models, these bold approaches remain at the forefront of discussions in the AI community, shaping both the competitive battleground and the future trajectory of AI capabilities.
Economic and Strategic Tradeoffs: Compute Costs, Inference Efficiency, and the AGI Timeline
Economic and strategic tradeoffs in the current AI landscape are driving decisions on both compute costs and inference efficiency, with significant implications for the AGI timeline. Companies face a constant balancing act: investing heavily in massive GPU clusters to train models versus optimizing inference to reduce ongoing operating costs. For example, breakthroughs in next-token prediction models and state-of-the-art post-training regimesâsuch as DeepSeekâs combination of reinforcement learning, instruction tuning, and preference fine-tuningâhighlight how even small improvements in compute efficiency can lead to notable performance gains. However, these gains come at a price. The speakers pointed out that the cost per question in inference can run into tens of dollars, underscoring the economic challenges in scaling these systems for widespread use.
On the training front, the debate between methodical, data-driven optimization and the bold, high-risk âYOLO runâ approach further illuminates the strategic tradeoffs. The lower GPU expenses that come with some post-training methodologies allow for more frequent experimental runs, which in turn can uncover unexpected performance breakthroughs. In one instance, DeepSeek built what they claimed was the largest GPU cluster in Chinaâa 10,000 A100 setupâto back both AI and quantitative trading models. Such scale not only reflects the commitment to pushing computational boundaries but also highlights the strategic gamble on compute investments that could translate into competitive advantage in AI research.
Inference efficiency plays an equally critical role, as models transition from raw compute-heavy training phases to real-time deployment. Advancements in transformer architectures, like optimizations surrounding memory bandwidth and the effective use of KV caches, show that the efficiency of internal data flows is now as important as raw floating-point operations (FLOPS). Companies like OpenAI and xAI are actively exploring these tradeoffs; even small differences in compute power can compound into breakthrough performance, potentially accelerating the journey toward AGI.
Speaking of AGI, the conversation framed the timeline as one influenced by these economic and technological factors. While some breakthroughs hint at an early form of general intelligence, experts caution that fully agentic, autonomous AIâa transformative ânuclear weapon momentâ in the fieldâmay not arrive until after 2030. In essence, the economics of compute, from massive training clusters to more efficient inference chips, directly affect how swiftly and effectively the AI community can navigate the long and complex path toward AGI.
This delicate balancing actâbetween compute costs, inference efficiency, and the strategic long game of AGIâillustrates the broader challenges the industry faces. As investments in hardware, cloud infrastructure, and innovative training methods continue to grow, the race to achieve AGI will likely be defined by those who manage to harmonize economic prudence with cutting-edge technical performance.
Geopolitical Tensions in Semiconductors: Export Controls, AI Chips, and Global Manufacturing
Geopolitical tensions in semiconductors have taken center stage amid fierce competition over advanced AI chips and global manufacturing capabilities. The U.S. has tightened export controls on its most powerful chipsâespecially those vital for AI, military applications, and data center operationsâto prevent China from quickly acquiring the technology. These restrictions not only target cutting-edge GPUs such as Nvidiaâs H800, H100, and H20, but also extend to key manufacturing tools like lithography, etching, and deposition equipment. By limiting access to these components, American policy makers aim to slow down Chinaâs progress, a strategy that recalls earlier measures which caused notable setbacks for companies like Huawei during its transition to 7-nanometer chips.
At the same time, China has responded by intensifying its own efforts. With massive state subsidies and aggressive investments, Beijing is doubling down on domestic chip production and AI hardware development. For instance, the discussion around DeepSeek highlights how Chinaâs ambitious GPU clustersâonce including a 10,000 A100 setup in 2021âserve dual purposes in both AI research and high-frequency trading, underscoring the nationâs determination to bridge the technological gap. While American companies like Nvidia and TSMC benefit from advanced manufacturing processes in the West, China appears poised to leverage both public support and innovation to challenge this dominance.
The global semiconductor supply chain has also been reshaped by the rise of the foundry model, championed by industry leader TSMC. Traditional approaches involving vertically integrated production are giving way to outsourcing for cost efficiency and scale. TSMC now acts as a critical backbone for both emerging specialized chip manufacturers and legacy companies, a shift that not only optimizes production costs but also exposes vulnerabilities when geopolitical tensions force restrictions on cross-border trade. This interdependence compounds the geopolitical stakes, as any disruption to the supply chain could have far-reaching economic impacts.
These challenges are further amplified by the ongoing evolution of AI chips. While newer generations such as the Nvidia H20 have seen modificationsâreducing FLOPS performance yet enhancing memory capacity and bandwidthâtheir capabilities remain crucial in AI training and inference, making them prime targets for export controls. This adjustment in chip performance, driven by U.S. policy, illustrates the complex trade-offs at play where technological projection meets strategic national interests. In this environment, every decision regarding export and manufacturing is interwoven with broader security and economic concerns, representing a dynamic frontier in global tech politics.
Leveraging the Semiconductor Supply Chain: TSMCâs Role and the Shifting Global Tech Balance
The semiconductor supply chain has become a critical lever in the global tech balance, with TSMC playing a central role. TSMCâs foundry model has transformed the industry by allowing companies to bypass the enormous capital required for building new fabsâa process that can cost as much as $30â40 billion per generation. Instead of investing in their own facilities, chip designers now outsource production to TSMC, benefiting from the companyâs scale and efficiency. This shift not only reduces expenses but also accelerates innovation, making it a vital cog in the AI and semiconductor ecosystem.
Recent discussions on the podcast highlighted how TSMCâs contributions extend beyond cost savings. By offering advanced manufacturing capabilities, TSMC has enabled tech giants to quickly scale up production, essential for powering everything from OpenAIâs supercomputing requirements to the massive GPU clusters pursued by companies like NVIDIA and xAI. As the geopolitical landscape grows increasingly complexâwith export controls and strategic tech bans influencing supply and demandâTSMCâs ability to meet advanced chip requirements positions it as a bridge between Western design innovation and the manufacturing prowess needed to build next-generation devices.
Furthermore, this dynamic is shifting the global tech balance. On one hand, Western companies rely on TSMCâs manufacturing expertise and economies of scale to stay competitive in fields like AI and high-performance computing. On the other, countries like China are pushing aggressively to evolve their domestic capabilities, sometimes subsidized by significant state investments, in response to export restrictions imposed by the United States. As one conversation participant noted, âTSMC now serves not only specialized chip manufacturers but even legacy companies that once depended on internal capacity,â underscoring how deeply integrated TSMC has become in the global semiconductor supply chain. This interconnectednessâbetween geopolitical strategy, technological innovation, and manufacturing efficiencyâis at the heart of the shifting tech balance, making TSMCâs role more pivotal than ever.
Mega GPU Clusters and Cooling Innovations: Overcoming Power and Interconnect Challenges
Mega GPU clusters have become a centerpiece in todayâs AI infrastructure, pushing the limits of power management and interconnect efficiency while demanding innovative cooling solutions. Leading tech companies are deploying clusters that number in the hundreds of thousands of GPUsâfor example, Elonâs cluster in Memphis reportedly includes around 200,000 GPUs (a mix of H100s and H200s), and competitors such as Meta, OpenAI, and Amazon are rapidly scaling their infrastructures, potentially reaching close to a million GPUs in the near future.
To support these vast arrays of processing units, engineers are overcoming several critical challenges. One major issue is power consumption: as GPUs compute vast amounts of data, transient power spikes can occur if weight synchronization isnât optimally overlapped with computation. This has led to creative software workarounds, such as a custom PyTorch operator humorously named âpytorch.powerplantnoblowup,â designed to simulate computation temporarily and mitigate dangerous energy surges.
Beyond the software techniques, the physical configuration of these data centers has seen significant innovation in cooling solutions. Traditional air cooling systems, which rely on heat pipes and fans, are proving insufficient for the sheer thermal load generated by modern GPUs. In response, pioneers like Elon Musk are employing large-scale liquid cooling systems. These systems use external water chillers and high-speed interconnects to cool GPUs more effectively, allowing them to be placed closer together. This proximity not only improves networking speeds but also enhances overall operational efficiency, making it possible to sustain the intensive compute required for both training and inference tasks.
Overall, the evolution of mega GPU clusters is a balancing act between raw computational power and the need for advanced cooling and power management solutions. The drive to maintain high-speed interconnects while managing escalating energy demands is at the heart of ongoing innovations, marking a significant step forward in the race to build the ultimate AI infrastructure.
Evolving AI Training Strategies: RLHF, Prompt Rewriting, and the Emergence of Chain-of-Thought
Evolving AI training strategies have seen significant innovation through techniques like reinforcement learning with human feedback (RLHF), prompt rewriting, and the emergence of chain-of-thought reasoning. In early deployments, RLHF often led models to produce outputs that felt overly constrained or even âdumb,â as the tuning process sometimes inadvertently limited the range of responses. However, as practitioners refined these approaches, the focus shifted toward creating systems that could both learn from human preferences and maintain high performance. For instance, while early RLHF iterations had a reputation for stunting a modelâs creative problem-solving ability, later adaptations began emphasizing reward models and preference fine-tuning to better align outputs with what users expect, striking a balance between safety and capability.
One notable example is the use of prompt rewriting. This technique involves transforming or refining user inputs before they reach the underlying model, a method that has shown promise in contexts such as enhancing image generation tasks as seen with Gemini. By rewriting prompts, models can generate descriptions or responses that are more accurate and contextually rich. However, the approach is not without its pitfallsâmissteps in prompt transformation can result in outputs that are obviously off-mark, highlighting that the art of rewriting requires precise calibration and continuous refinement.
The evolution of chain-of-thought reasoning adds another fascinating layer to AI training. Unlike models that only provide a final answer, systems incorporating chain-of-thought openly display their internal reasoning processâa sequential unfolding of thought that mirrors human reflective problem-solving. This approach has been likened to the narrative depth found in literary works such as "Ulysses" or "Finnegans Wake," offering a glimpse into the modelâs deliberative journey. Such transparency not only builds trust but also allows researchers to identify and address any logical missteps within the modelâs reasoning pipeline.
These strategies are underpinned by iterative improvements in learning techniques. By combining trial-and-error reinforcement learning with traditional imitation learningâwhere models learn from both human annotations and outputs produced by more powerful systemsâthe field has made leaps in overcoming the limitations inherent in any single approach. As Andrej Karpathy and others have noted, this blend of techniques is fostering more robust and flexible AI that can better navigate the complexities of natural language and decision-making.
Together, RLHF, prompt rewriting, and chain-of-thought methodologies represent a dynamic shift in AI training strategies. By continuously evolving these methods, researchers are not only enhancing performance but also moving toward systems that offer greater transparency and alignment with human thought processesâa vital step as we push closer to more general forms of artificial intelligence.
Ethical and Legal Frontiers: Supervised Fine-Tuning, Distillation, and Data Ownership Debates
The discussion around ethical and legal frontiers in AI training is complex and multifaceted, reflecting debates over supervised fine-tuning, model distillation, and data ownership. At the heart of this conversation lies the question of how AI developers can build on the extensive range of freely available internet data while navigating the nuanced constraints imposed by licenses and terms of service. For example, while many models are fine-tuned using completions provided by human annotators or even outputs from larger models like the rumored âGPTâ5â or âClaude 3,â questions arise about the legitimacy of training on these outputs. Critics argue that the practice of distillationâessentially âcopyingâ or repurposing outputs from dominant systemsâposes ethical dilemmas, especially when such outputs originate from companies with strict usage conditions.
This debate is particularly acute given the industry's precedents. Some voices in the discussion note that early-stage models were bootstrapped using data generated by established systems, highlighting a precedent for such practices. However, while open-source models have thrived on extensive, publicly available datasets, the current environment is more contentious, with major players like OpenAI enforcing terms that seemingly prevent competitors from leveraging their proprietary outputs. This situation creates a puzzling dynamic: on one hand, AI companies credit the influence of ubiquitous internet contentâwhich often originates from large modelsâand on the other, they strictly regulate direct reproduction of outputs through contractual agreements.
Legal nuances further complicate the landscape. Jurisdiction-specific examples, such as Japanâs more lenient copyright laws for model training, illustrate that what is permissible in one region might be contested in another. The ambiguity surrounding terms like âcompetitorâ adds another layer of complexity, leaving room for interpretation that can potentially be exploited. Such uncertainties not only bring ethical questions into sharp focus but also raise fears of unintended legal consequences. The contrast between the methodical nature of supervised fine-tuning and the more exploratory reinforcement learning (RL) approach exemplifies how technical choices in training are deeply intertwined with legal and ethical considerations.
Overall, the dialogue suggests that while supervised fine-tuning and distillation offer promising pathways to refine AI behavior, they also force a reevaluation of data ownership and fair use in an era where back-and-forth borrowing of ideas and outputs is becoming the norm. As AI research pushes forward at breakneck speed, the need for clear guidelinesâones that address both the innovative spirit of the field and the rights of content creatorsâis more pressing than ever.
Human Impact and the Future of AGI: Agentic Capabilities, Global Dynamics, and Societal Change
The evolution of AGI and its increasingly agentic capabilities is poised to reshape global dynamics and the fabric of society. As models transition from merely predictive engines to autonomous agents capable of independent reasoning and decision-making, the human impact grows exponentially. For example, discussions on the Lex Fridman Podcast emphasized that while today's language models demonstrate impressive general intelligence, the next phase of AI is expected to function autonomously, potentially driving complex tasks without direct human intervention. This shift is seen by some experts as a turning pointâsometimes even compared to a ânuclear weapon momentââwhere autonomous systems could either unlock unprecedented opportunities or introduce significant security risks.
At the heart of these developments is the interplay between massive computational power and innovative training techniques such as reinforcement learning from human feedback (RLHF) and chain-of-thought strategies. The transparency and technical depth provided by initiatives like DeepSeekâs detailed training documentation have fostered a better understanding of how agentic capabilities emerge. By meticulously refining models down to their CUDA-level operations and employing advanced post-training methods like instruction tuning and reinforcement learning, researchers have aimed to align AI outputs more closely with human expectations. Such advancements not only improve performance but also lay the groundwork for systems that can operate with a higher degree of autonomy.
Global dynamics are also being shaped by these technological leaps. The ongoing debate over export controls on advanced AI chips and the race between hardware giants like NVIDIA and emerging competitors in China illustrate how intertwined AGI development has become with geopolitical strategy. Export restrictions and the strategic build-up of mega GPU clustersâfrom DeepSeek's early use of massive GPU setups to Elon Muskâs liquid-cooled clustersâdemonstrate that the future of AGI is as much about technology as it is about international power plays. As nations push for self-reliance in semiconductor production and AI capabilities, the institution of policies to manage these advancements becomes critical, influencing global economic and political stability.
Finally, at the human level, the amplification of individual impact through AGI offers both promise and peril. In one part of the discussion, speakers noted that while AGI can magnify human achievements and contribute to significant reductions in societal suffering, it can also exacerbate issues like misinformation, election interference, and even conflict if its autonomous actions are misdirected. The notion that âhumans are not just social animals, but self-domesticated apesâ served as a reminder of our complex relationship with technologyâa balance between leveraging intelligence to enhance our lives and ensuring that unforeseen agentic behaviors remain under ethical and regulatory control. As Richard Feynman famously observed, ânature cannot be fooled,â underscoring the necessity to ground our ambitions in objective reality as we approach this transformative era.